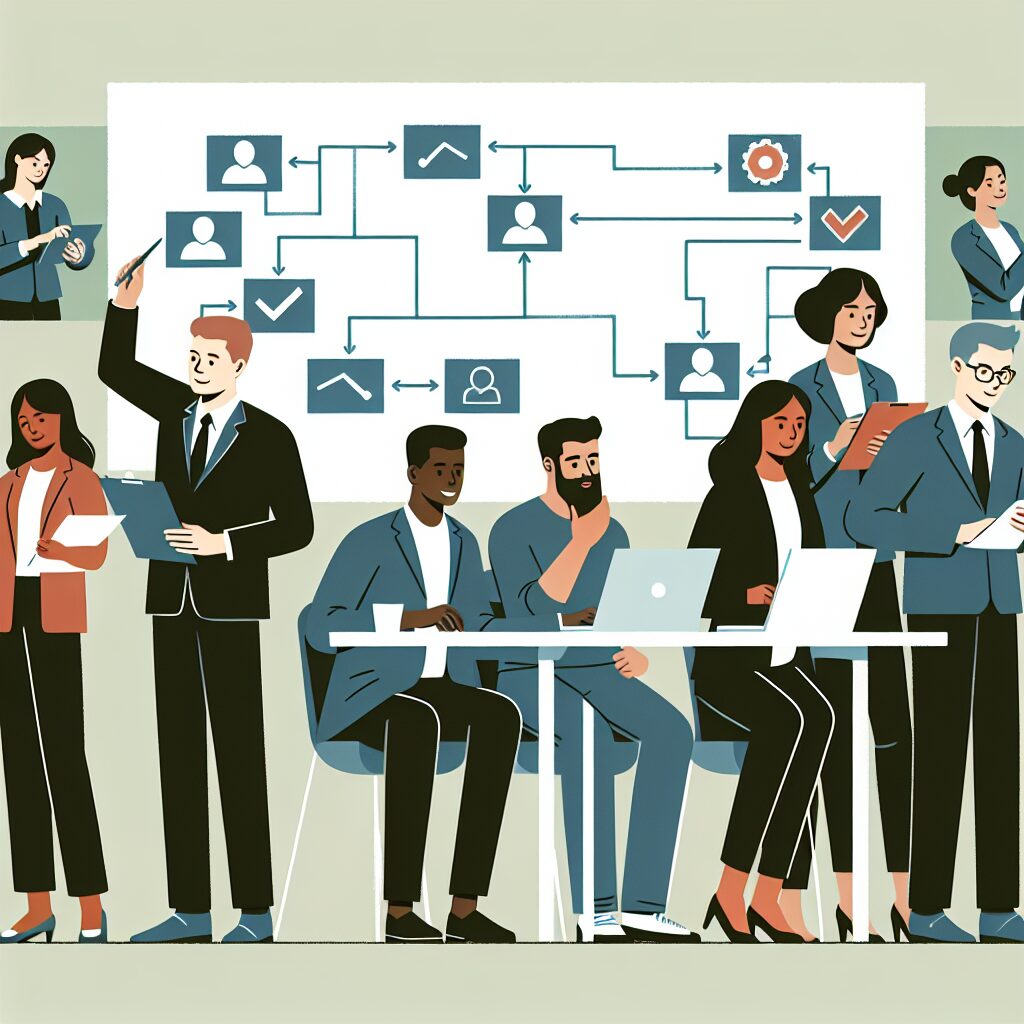
How to Sustain an AI Advantage: Beyond the Pilot Project
For many businesses, the allure of Artificial Intelligence (AI) has translated into a flurry of pilot projects. These initial forays often deliver promising results, demonstrating the potential for increased efficiency, cost reduction, or improved customer experience. However, the journey from pilot project to sustained competitive advantage is fraught with challenges. Success in the initial phase is only the first step; true differentiation comes from strategically evolving your infrastructure, refining your metrics, and nurturing a culture that embraces continuous AI development.
The initial appeal of AI is often its ability to automate repetitive tasks or provide predictive insights based on existing data. These isolated successes can be misleading if not integrated into a broader, more sustainable AI strategy. Without careful planning, businesses risk creating a patchwork of disconnected AI applications that offer limited long-term value. Moving beyond this pilot phase requires a fundamental shift in perspective, transitioning from viewing AI as a tactical tool to seeing it as a strategic asset.
Building a Robust AI Infrastructure:
The foundation of any sustainable AI advantage lies in a robust and scalable infrastructure. This goes beyond simply selecting a few cloud-based AI services. It requires a holistic approach that addresses data management, compute resources, and the integration of AI into existing business processes.
- Data as a Strategic Asset: High-quality, accessible data is the lifeblood of any AI system. Organizations must prioritize data governance, ensuring data accuracy, consistency, and compliance with relevant regulations. This includes establishing clear data ownership, implementing robust data security protocols, and developing standardized data formats to facilitate seamless integration across different AI applications. Furthermore, a centralized data repository, or data lake, is crucial for providing a single source of truth for AI models. Investing in data preparation tools and techniques is essential for cleaning, transforming, and enriching data to maximize its usefulness for AI algorithms.
- Scalable Compute Resources: AI models, especially deep learning algorithms, require significant computational power for training and inference. Organizations must ensure they have access to sufficient compute resources, whether through cloud-based platforms, on-premise infrastructure, or a hybrid approach. Scalability is key; as AI adoption expands, the demand for compute resources will inevitably increase. Consider utilizing specialized hardware, such as GPUs or TPUs, to accelerate AI workloads and improve performance.
- Seamless Integration: AI shouldn’t exist in a vacuum. To realize its full potential, AI must be deeply integrated into existing business workflows and systems. This requires careful planning and execution, ensuring that AI models can seamlessly interact with other applications and data sources. APIs (Application Programming Interfaces) play a crucial role in enabling this integration, allowing different systems to communicate and exchange data effectively. Furthermore, consider adopting a microservices architecture to build modular and scalable AI applications that can be easily integrated into existing infrastructure.
Refining Metrics for Continuous Improvement:
While initial pilot projects often focus on simple metrics like accuracy or cost savings, sustaining an AI advantage requires a more nuanced approach to measurement. Businesses must define Key Performance Indicators (KPIs) that accurately reflect the business value generated by AI and track progress over time.
- Beyond Accuracy: While accuracy is important, it’s not the only metric that matters. Consider factors like precision, recall, F1-score, and AUC (Area Under the Curve) to gain a more comprehensive understanding of model performance. Furthermore, evaluate the impact of AI models on downstream business processes. For example, if an AI model is used to predict customer churn, measure the actual reduction in churn rate resulting from the model’s predictions.
- Business-Oriented KPIs: Align AI metrics with broader business objectives. Instead of focusing solely on technical performance, track metrics that directly impact revenue, profitability, customer satisfaction, or operational efficiency. For example, measure the increase in sales attributed to AI-powered personalized recommendations, the reduction in operating costs resulting from AI-driven automation, or the improvement in customer satisfaction scores due to AI-enabled customer support.
- Continuous Monitoring and Evaluation: AI models are not static; their performance can degrade over time as the underlying data changes. Implement a robust monitoring system to track model performance in real-time and identify potential issues. Regularly retrain models with new data to maintain their accuracy and relevance. Furthermore, periodically evaluate the impact of AI models on business outcomes and make adjustments as needed to optimize performance and maximize value. A/B testing and champion/challenger methodologies can be powerful tools for identifying opportunities for improvement.
Nurturing a Culture of AI Innovation:
Technology alone is not enough to sustain an AI advantage. Organizations must cultivate a culture that embraces continuous learning, experimentation, and collaboration. This requires fostering a mindset where employees are encouraged to explore new AI applications, experiment with different techniques, and share their knowledge and insights.
- Democratizing AI: Empower employees across different departments to participate in AI initiatives. Provide them with access to AI tools and resources, and offer training programs to enhance their AI skills. Encourage citizen data scientists to develop and deploy their own AI models, under appropriate governance and security controls. This democratization of AI can unlock new opportunities for innovation and drive broader adoption across the organization.
- Cross-Functional Collaboration: AI projects require collaboration between data scientists, engineers, business analysts, and domain experts. Foster a collaborative environment where these different stakeholders can work together effectively to define project requirements, develop AI solutions, and deploy them into production. Establish clear communication channels and encourage open dialogue to ensure that everyone is aligned on project goals and objectives.
- Embrace Experimentation: Encourage employees to experiment with new AI techniques and technologies. Create a safe space for failure, where employees are not penalized for trying new things and learning from their mistakes. Organize hackathons and innovation challenges to generate new ideas and foster a culture of creativity.
- Leadership Commitment: Ultimately, the success of any AI initiative depends on the commitment of senior leadership. Leaders must champion AI adoption, allocate resources to AI projects, and create a culture that values innovation and experimentation. This includes clearly communicating the organization’s AI strategy, setting realistic expectations, and celebrating successes.
Sustaining an AI advantage is not a one-time effort; it’s an ongoing journey that requires continuous investment, learning, and adaptation. By building a robust infrastructure, refining your metrics, and nurturing a culture of AI innovation, you can transform early AI wins into a lasting competitive advantage.
To navigate the complexities of AI strategy and leadership, consider the insights offered in our eBook, The Invisible Chief AI Officer: Why Many Businesses Need a Leader They May Not See. Learn how to effectively integrate AI into your organization and unlock its full potential. Purchase your copy today at https://store.mymobilelyfe.com/product-details/product/the-invisible-chief-artificial-intelligence-officer.
Recent Comments